The RWTH Aachen - University of Melbourne Joint PhD Group (RUM) was established in 2019 with a focus on research looking at the broad theme of energy. The group includes joint PhD candidates, early career researchers, and senior academics who collaborate on projects ranging from renewables to engine performance.
Joint PhD candidates participating in the RUM Group are a part of a group investigating significant scientific challenges, jointly supervised and mentored by academics from both RWTH Aachen and Melbourne. These candidates spend time researching in both Germany and Australia, have access to facilities and professional development opportunities at both institutions, and benefit from being part of the RUM network.
Our partner: RWTH Aachen
RWTH Aachen, or Rheinisch-Westfälische Technische Hochschule, is a German research university renowned for its science and engineering disciplines. The world’s first wind tunnel and particle accelerator were both developed at RWTH Aachen. RWTH Aachen is one of Germany's eleven "Universities of Excellence" - leading universities recognised for their outstanding research capabilities.
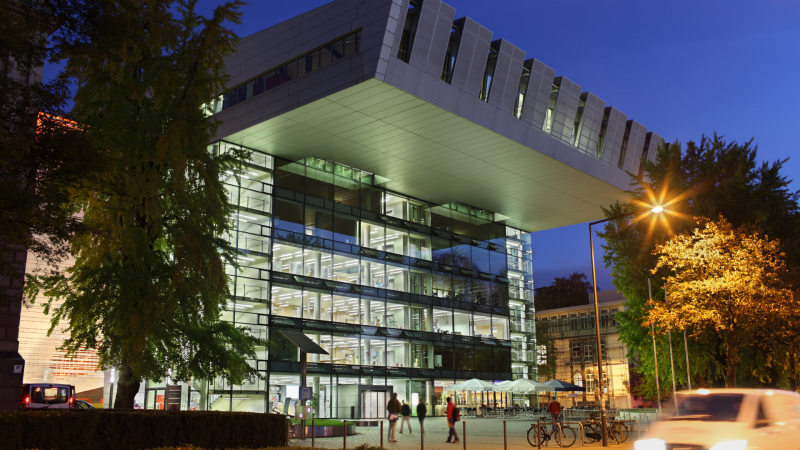
The opportunity to be a RUM joint PhD candidate has been a life-changing experience.Weiqi Sun RWTH Aachen - Melbourne Joint Training Group candidate
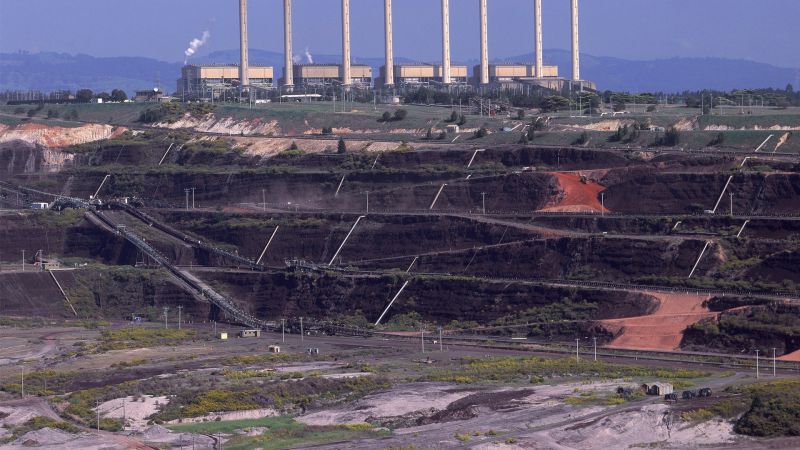
Project spotlight
Optimising the reuse of decommissioned coal mines
What should we do with coal mines once they’re decommissioned? Australia and Germany are both coal mining intensive countries, and this question continues to be a point of contention for local officials, residents and technical experts. This project aims to use numerical modelling tools to evaluate the best method of backfilling (the process of reusing or replacing the soil used during excavation) open cast coal mines. Using decommissioned coal mines effectively and innovatively will help meet emission-reduction targets and contribute to the fight against climate change.
Meet our academic lead
Professor Sandra Kentish is the academic lead of the RWTH Aachen-Melbourne (RUM) Joint PhD program. She is Head of the School of Chemical and Biomedical Engineering and a Redmond Barry Distinguished Professor at the University of Melbourne. Professor Kentish has broad interests in industrial separations, particularly the use of membrane technology for energy, food and water applications. She was selected as one of Australia's Most Innovative Engineers by Engineers Australia in 2017 and as a Woman of Influence by the Australian Financial Review in 2018.
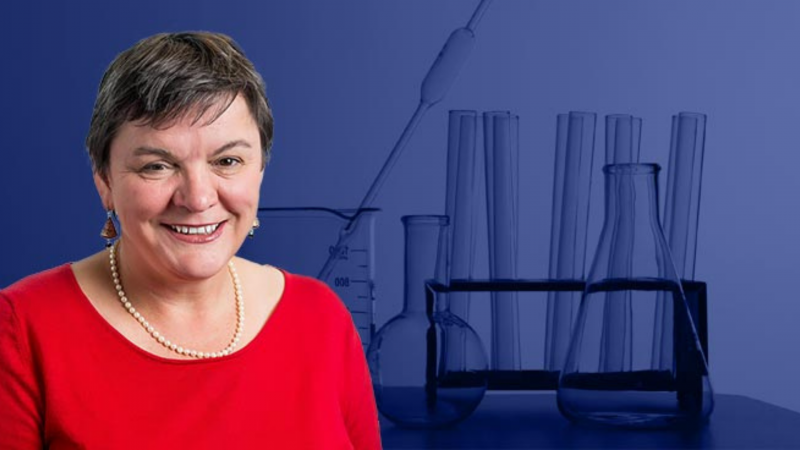
First published on 5 September 2022.
Share this article
Related items
-
International PhD opportunities
Discover the fully funded Joint PhD opportunities that are currently available with universities and research institutions around the world.
-
Find a supervisor or research project
Discover how to find a supervisor and learn how they can support your graduate research, or search available research projects.
-
Research degrees
Become a graduate researcher at the University of Melbourne.
-
Your study experience
Discover what it's like to be a graduate researcher. Find out about University life, support services, and opportunities for skills development.